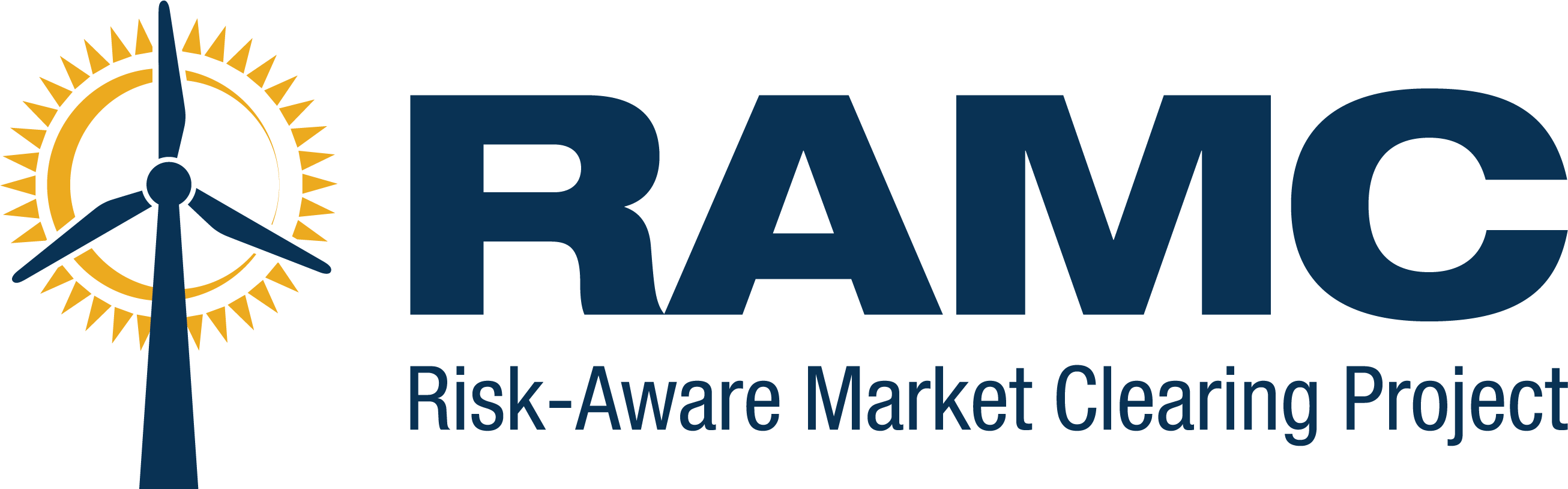
The increasing role of renewable energy sources is challenging grid operations, which have traditionally relied on highly predictable load and generation. Future grid operations must balance generation costs and systemlevel risk, shifting from deterministic to stochastic optimization and risk management. The Risk-Aware Market Clearing (RAMC) project will provide a blueprint for an end-to-end, data-driven approach where risk is explicitly modeled, quantified, and optimized, striking a tradeoff between cost and system-level risk minimization. The RAMC project focuses on challenges arising from increased stochasticity in generation, load, flow interchanges with adjacent markets, and extreme weather. RAMC addresses these challenges through innovations in machine learning, sampling, and optimization. Starting with the risk quantification of each individual asset obtained from historical data, RAMC learns the correlations between the performance and risk of individual assets, optimizes the selection of asset bundles, and quantifies the system-level risk.
THE CHALLENGE //
The RAMC project focuses on the challenges faced by the Midcontinent Independent System Operator (MISO), a not-for-profit organization that delivers safe, cost-effective electric power to 42 million customers across 15 U.S. states and the Canadian province of Manitoba. MISO’s power grid includes 71,800 miles of transmission lines and a generation capacity of 177,760 MW for a peak summer system demand of 127,125 MW. Its current generation mix includes 43% gas, 30% coal, 17% renewables, and 8% nuclear. The challenge of the RAMC project is to help MISO manage increased uncertainty as its portfolio evolves to include more renewable energy sources in the generation mix. MISO has set ambitious targets for renewable energy, including reaching 32% of the generation mix by 2030. Given the intermittency of wind and solar energy; the increasing prediction errors; the new types of risk and overall risk growth; and the uncertainty around flow interchanges in adjacent markets and extreme weather, it is becoming abundantly clear that a paradigm shift is needed in how the grid is operated, moving from deterministic optimization to market clearing algorithms that directly capture and reason about risk and uncertainty.
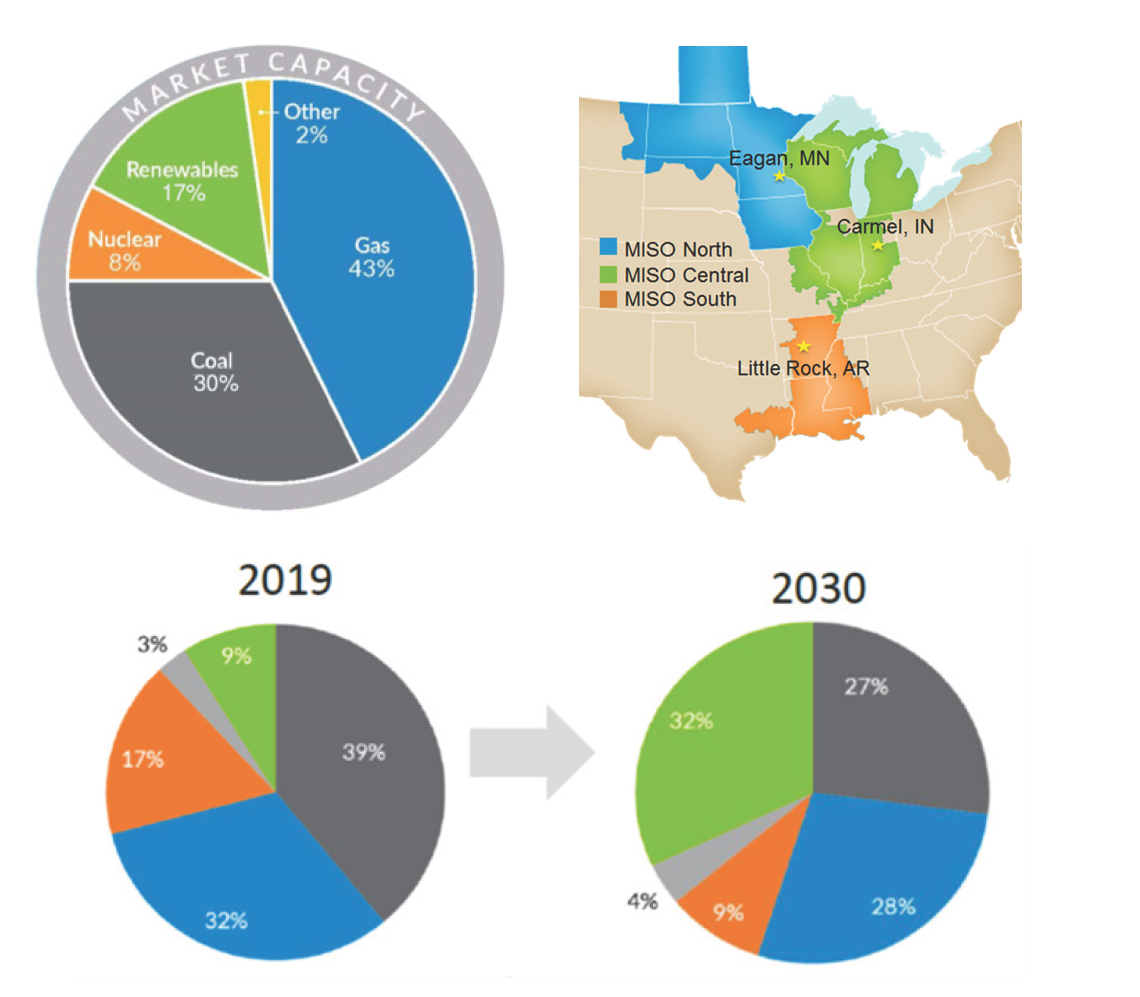
Historical Projected MISO-Wide Generation Mix
END-TO-END RISK MANAGEMENT //
The goal of RAMC is to design and implement an end-to-end approach to risk management, starting from seven-day forward reliability assessment commitment (FRAC), moving to the security-constrained unit commit (SCUC), and culminating in the look-ahead commitment (LAC) and the real-time security-constrained economic dispatch (RT-SCED). These market-clearing models operate at different temporal scales and levels of uncertainty, but their decisions are propagating in time. It is thus critical to adopt risk and uncertainty holistically and to use appropriate techniques for each stage.
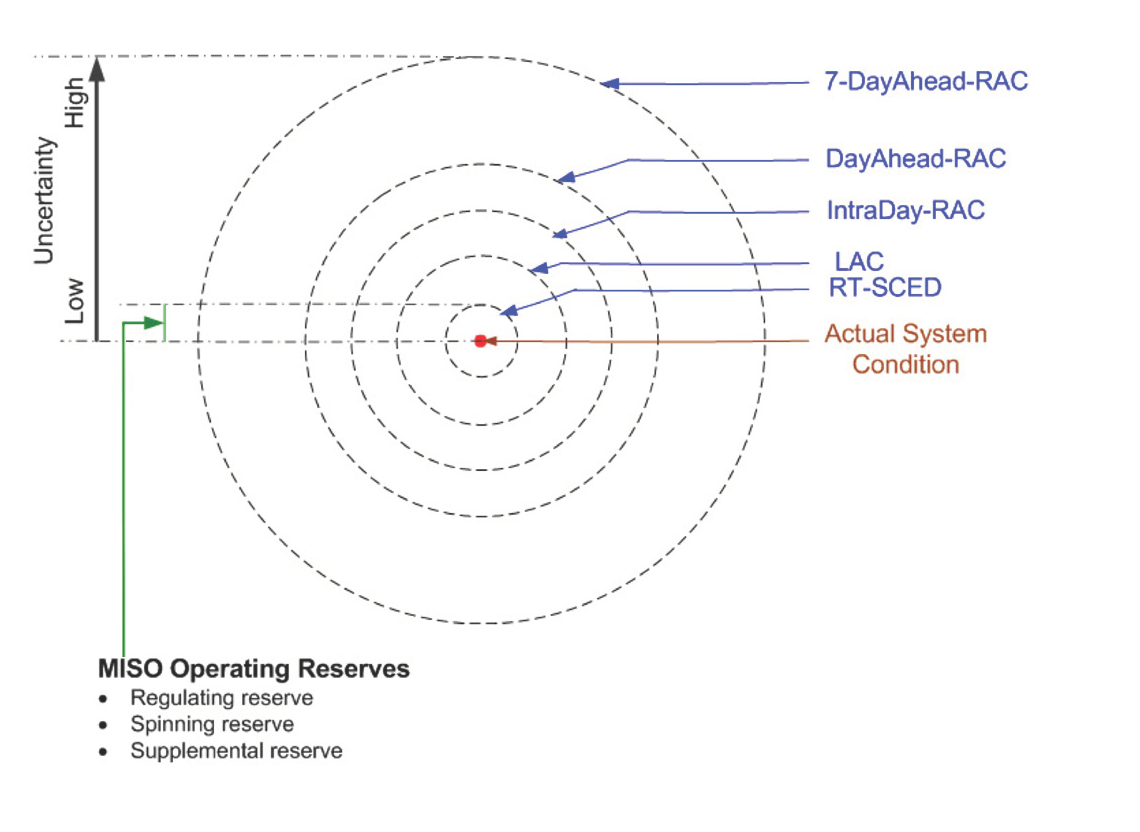
MISO Operating Reserves
THE RAMC APPROACH //
RAMC will focus on five main components to address the challenges faced by MISO and develop an end-to-end approach to risk-aware market clearing. They are (1) uncertainty quantification; (2) dimensionality reduction; (3) system-level risk assessment; (4) stochastic programming; and (5) machine learning. They will be integrated in a multi-year high-fidelity simulation to demonstrate the benefits of the proposed approach in terms of a wide range of efficiency and risk metrics. The approach is summarized in Figure 1.
- Uncertainty Quantification: to quantify the uncertainty coming from renewable energy more precisely, exploiting spatial and temporal correlations. Figure 2 and Figure 3.
- Dimensionality Reduction: to reduce the number of scenarios needed to perform system-level risk assessment and stochastic optimization.
- Risk-Level System Assessment: to provide precise risk measures for the system to inform operators and market clearing algorithms, using concept from the financial industry.
- Stochastic Optimization: to develop a new generation of market-clearing algorithms for the FRAC, LAC, and RT-SCED, moving from deterministic optimization to robust and stochastic optimization.
- Machine Learning: to develop optimization proxies to run deterministic and stochastic algorithms with similar accuracy and orders of magnitude speed-ups in efficiency.
- Multi-Year High-Fidelity Simulation: the proposed approach will be validated in a counterfactual study to demonstrate the benefits of the approach.
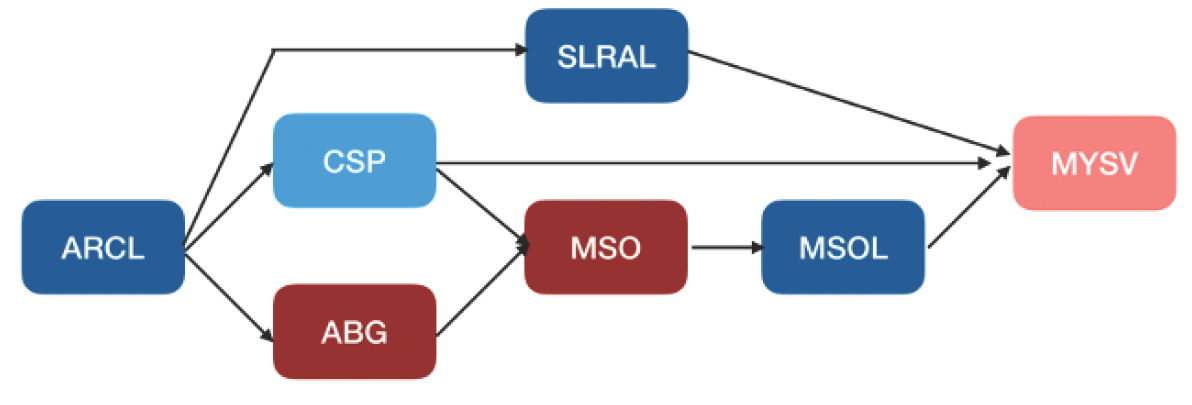
Figure 1. The Overall Organization of RAMC
ARCL: Asset Risk Correlation Learning; CSP: Conditional Sampling Procedure; ABG: Asset Bundling Generation; SLRAL: System-Level Risk Assessment; MSO: Multistage Stochastic Optimization; PSOL: Power System Optimization Learning; MYSV: Multi-year Scenario Validation; VCS: Visualization of Counterfactual Studies
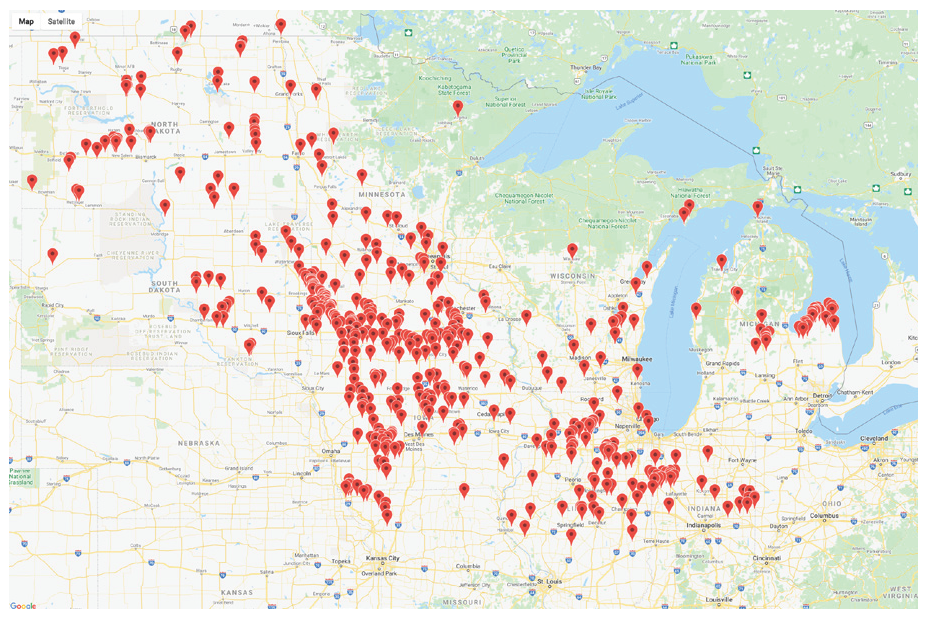
Figure 2. Wind farms in MISO North
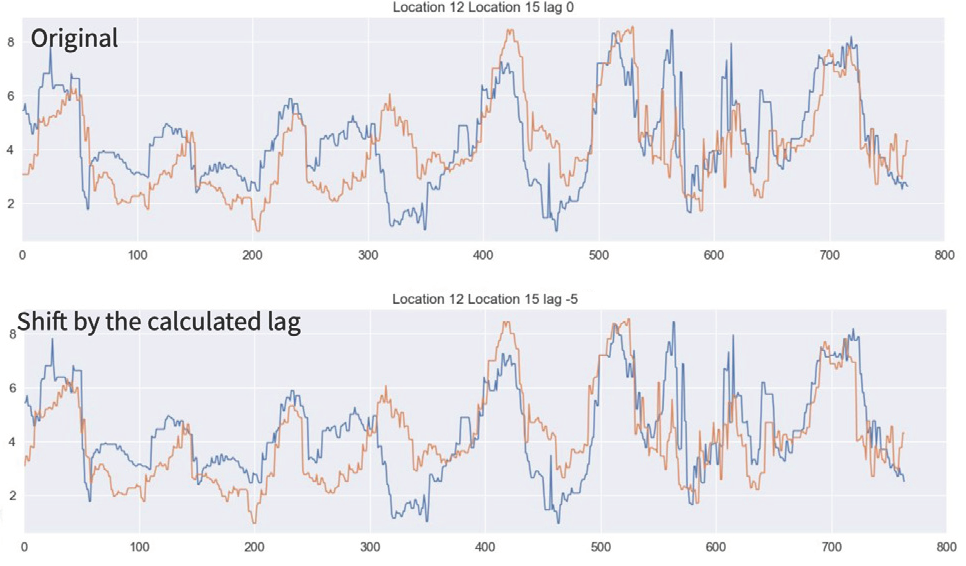
Figure 3. Exploiting wind correlations for asset bundling
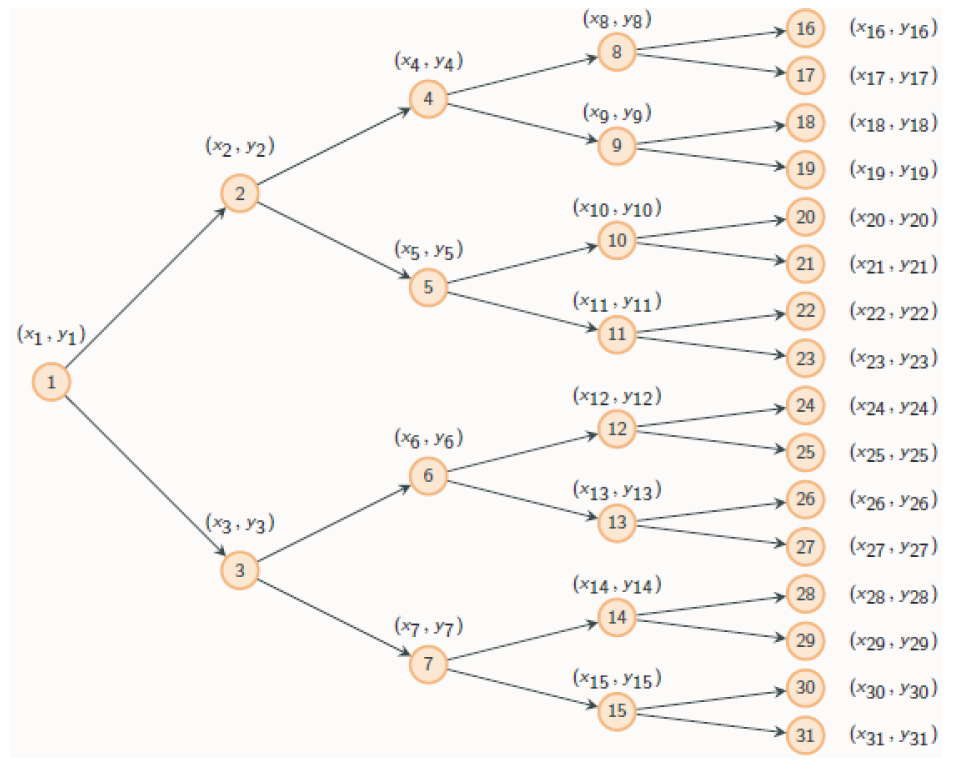
Figure 4. Stochastic Dual Dynamic Integer Programming
PILOT STUDY //
RAMC is also engaging in an ambitious pilot project that considers an Extended LAC, i.e., the LAC extended from three to six hours before actual system operations. The Extended LAC is particularly challenging due to the significant uncertainty about renewable generation, interchanges, and loop flows. It also deals with decisions on when to commit generators in real time, that have substantial impact on both risk and costs. It thus represents an ideal case study to demonstrate the benefits of risk-aware market clearing as enabled by uncertainty quantification, risk assessment, stochastic optimization, and machine learning. The challenges raised by the Extended LAC are not unique to MISO: They are also present in other ISOs, highlighting its need and potential benefits.
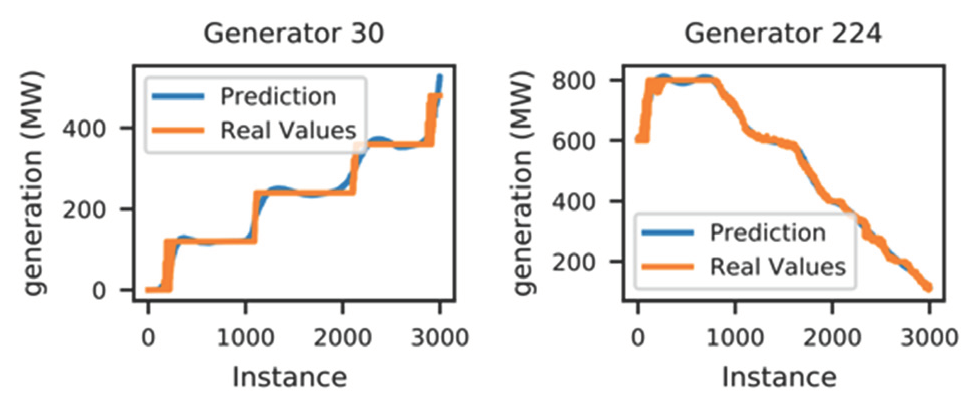
Machine Learning for Security-Constrained Economic Dispatch
Team
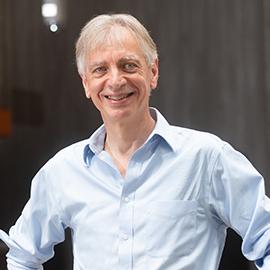
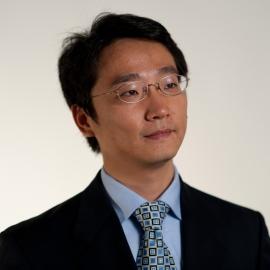
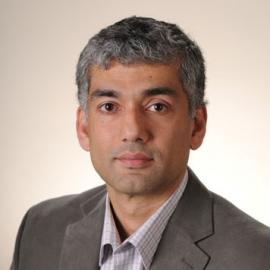
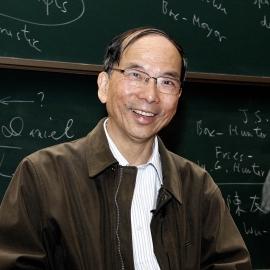